Artificial Intelligence (AI) is reshaping various facets of our world, particularly in agriculture.
Advanced technologies are now leveraged to predict crop yields, providing numerous benefits to farmers and the agriculture industry as a whole.
Accurate forecasts of agricultural production are crucial for strategic planning and risk management.
More than ever, farmers are using AI to attain precision and efficiency in their operations.
From real-time plant health monitoring to optimized resource utilization, AI is playing an integral role.
This post will dig in into how this technology is revolutionizing the agriculture sector, specifically in predicting crop yields.
- AI improves irrigation methods via weather prediction functionality.
- AI enhances pest detection and control for optimal yield.
- Accurate soil analysis is feasible through AI-driven techniques.
- AI provides real-time crop growth monitoring and weed detection.
- Optimal harvest time and yield forecasting achievable using AI models.
While the initial insights shared highlight the impact of AI on crop yield prediction, there are additional layers to this subject that we are going to explore.
In the upcoming sections, we will dig deeper into how AI is leading innovations in areas such as crop disease prediction and precision farming.
Unveiling these concepts will equip you with a broader understanding of the role AI plays in modern agriculture.
Stay tuned as we continue to unravel these intriguing developments within agricultural technology.
Contents
- Ways Ai Is Transforming Crop Yield Prediction
- 1. Optimize irrigation through AI-based weather prediction
- 2. Enhance Pest Detection and Control via AI
- 3. Machine Learning for Precision Fertilization
- 4. Accurate AI-driven soil analysis for better yields
- 5. Predictive Analytics for Crop Diseases
- 6. Real-time crop growth monitoring with AI algorithms
- 7. Automating Weed Detection Using AI
- 8. AI allows comprehensive field imagery and analysis
- 9. Optimizing Harvest Time through AI Models
- 10. Machine Learning Models for Crop Yield Forecasting
- The Bottom Line
Ways Ai Is Transforming Crop Yield Prediction
1. Optimize irrigation through AI-based weather prediction
In Short: AI-based weather prediction in agriculture enhances irrigation efficiency and boosts crop yields by accurately forecasting local weather conditions. Tech companies are developing AI systems that analyze climate data to ensure optimal water usage, predict pest attacks, and nutrient deficiencies, leading to sustainable and profitable farming practices.
Rapid advancements in technology have paved the way for novel applications in agriculture, one of which is AI-based weather prediction.
Artificial Intelligence (AI) has been making profound inroads when it comes to optimizing irrigation, enhancing the efficiency of water usage, and ultimately boosting crop yields.
Historically, farmers have primarily relied on their empirical knowledge and intuition to predict weather conditions and accordingly irrigate their fields.
However, factors such as climate change and unpredictable weather patterns have pummeled these traditional techniques.
AI-based weather prediction for irrigation control comes as a far more efficient, reliable, and scalable alternative.
Using machine learning algorithms, gigantic data sets of historical and present weather conditions can be analyzed to generate accurate and localized weather forecasts.
These forecasts then assist in automating irrigation processes, ensuring optimal water usage at the right time.
Its worth noting some integral aspects related to AI’s use in irrigation:
- AI has the potential to significantly reduce wasteful water usage.
- It ensures plant health by providing adequate water at the right time.
- Such precision, in turn, directly results in more abundant crop yields.
Various tech companies and agritech startups have started incorporating AI technology in their products to aid farmers in managing their irrigation systems.
These intelligent systems, composed of sensors and AI algorithms, provide real-time data about the soil’s moisture levels, temperature, and other climatic conditions.
This data is then processed through machine learning models to predict the optimal time and quantity for irrigation, taking the guesswork out of the equation.
Such predictive analytics help not just with irrigation, but can also forecast possible pest attacks, plant diseases, nutrient deficiencies, and more.
Moreover, as the system collects and learns from more and more data, its predictions become increasingly accurate and reliable.
There’s no doubt that AI-based irrigation backed by weather prediction is a revolution in sustainable farming practices.
In fact, with the conquest of AI in irrigation systems, we are setting a firm stride towards agriculture that is resource-efficient, sustainable, and profitable.
However, it’s important to remember that the implementation and success of such predictive systems are dependent on factors such as technology accessibility, farmer education, and data privacy.
2. Enhance Pest Detection and Control via AI
In Short: AI technology is overhauling traditional pest control methods in farming, offering predictive, precision-based solutions that are efficient, economical, and environmentally friendly. Utilizing AI capabilities not only improves pest detection but could potentially stem infestations through early preventive measures, leading to increased crop yield and minimized usage of pesticides.
Pest management is truly crucial for successful farming, and the advent of AI technology is proving a game-changer in this sphere.
Traditional methods of pest control involve extensive labor, time, and aren’t always effective.
The incorporation of AI into this domain is beginning to revolutionize pest detection and control, providing simpler, quicker, and more efficient solutions.
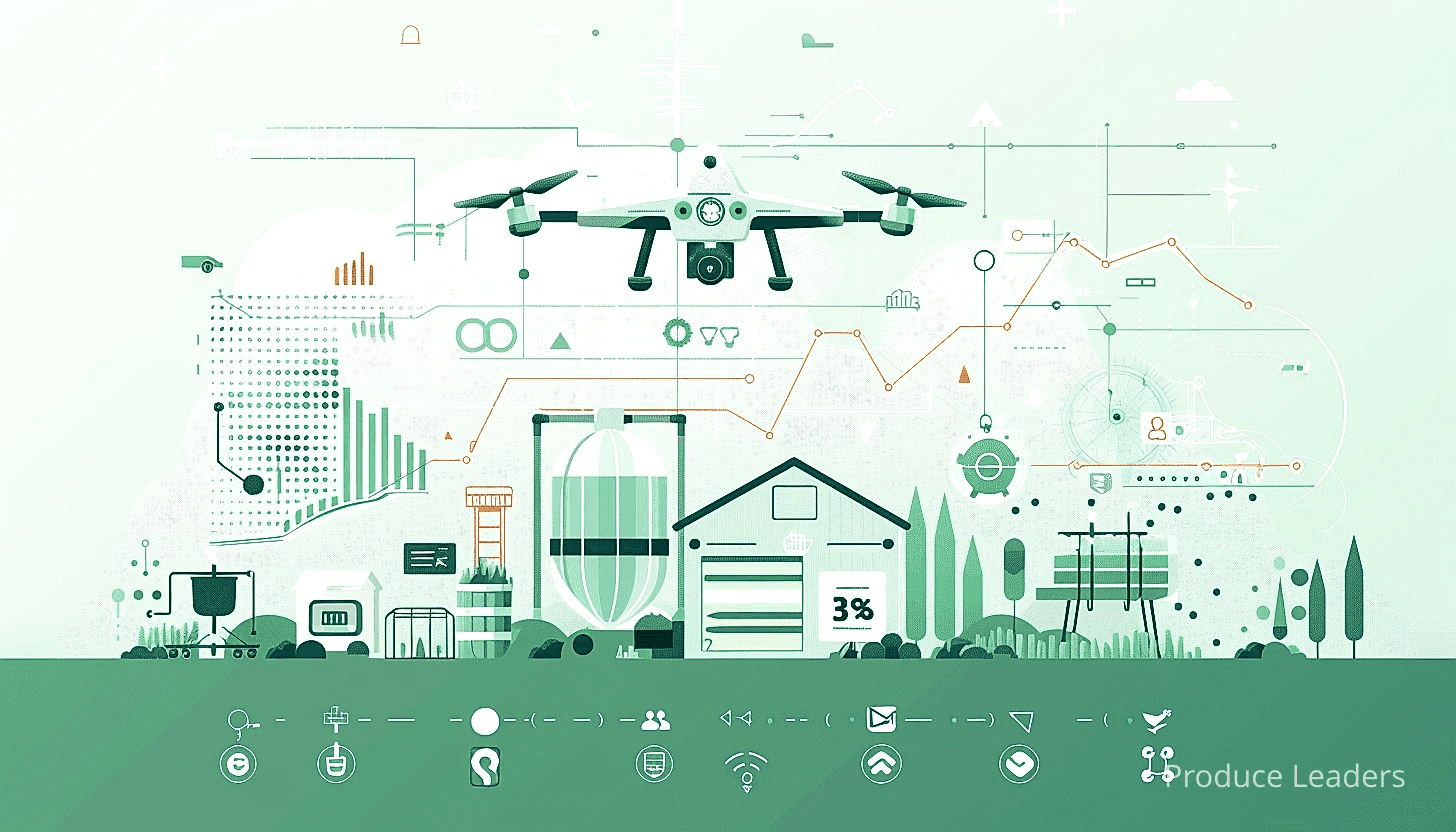
For instance, AI-powered drones armed with high-resolution cameras are now able to scan large swathes of farmland to detect pest infestations.
The imagery captured by these drones is then analyzed through deep learning algorithms for identification of specific pests and pest-damaged crops.
The speed and accuracy of such AI-driven pest detection far surpasses manual methods.
Moreover, AI can help not only detect but also predict possible pest attacks.
By feeding historical weather and crop data into predictive AI models, farmers can be alerted of potential pest attacks in advance.
Here are some benefits that can be realized through the integration of AI in pest detection and control:
- Decreased reliance on pesticides: Intelligent pest detection and prediction reduces the need for excessive pesticide application, thereby reducing costs and environmental impact.
- Increased crop yield: By addressing pest issues promptly and accurately, crop health can be optimized leading to a superior yield.
- Preventive action: Through AI’s predictive ability farmers can take early action to prevent or minimize pest damage. This potential to predict and preempt pest infestations is transformative.
- Reduced labor costs: AI-driven pest control reduces the time and labor spent on traditional pest management methods. This indirectly contributes to cost efficiency and productivity.
Moving forward, AI holds a strong potential to recognize new pests and diseases that haven’t been manually documented before, tapping into its machine learning capabilities.
Over time, these machine learning models will continue to evolve and improve on their own, further enhancing their precision and response time.
Research is being carried out to combine AI and biology for creating biocontrols for pests, using natural predators instead of chemicals or pesticides.
This is an exciting area of research and is expected to offer innovative solutions to pest control in the future.
Overall, the inclusion of AI in pest detection and control is helping reduce costs, save time, increase efficiency, and maximise crop yield.
As researchers continue to innovate and fine-tune AI technology, the future of pest management in agriculture appears brighter than ever.
3. Machine Learning for Precision Fertilization
In Short: Machine learning is revolutionizing agriculture through precision fertilization, allowing detailed analysis of field data to accurately predict crops’ nutrient requirements. This technology not only maximizes crop yields, but also protects the environment, enhances efficiency, and optimizes farm expenditures, fostering sustainable and cost-effective farming.
One of the significant ways AI is enhancing modern agriculture is through innovative approaches like precision fertilization, powered by machine learning techniques.
Every farmer understands the important role fertilizers play in plant growth and development. Nonetheless, optimal fertilization is often tricky to achieve, especially regarding identifying the perfect amount and type for specific crops and field conditions.
To address this challenge, researchers have turned to machine learning, which offers an intelligent way to deeply analyze field data, predict a crop’s nutrient requirements, and apply the necessary fertilization.
This approach is not only enabling farmers to achieve maximum crop yields, but it also conserves the environment by reducing the risk of fertilizer overuse, which often results in harmful pollutant runoff.
Machine learning works in precision fertilization by leveraging predictive analytics on vast historical crop data and continually learning from it, ensuring the most accurate fertilizer recommendations are made to the user.
These algorithms can consider a myriad of factors, such as soil type, crop genotype, growth rate, and weather forecasts, to optimize fertilization strategies.
Here are some of the primary ways that machine learning aids in precision fertilization:
- Predictive Analytics: AI systems can analyze historical data to predict the necessary fertilizers for specific crops within a particular microclimate or soil type.
- Real-time Feedback: Through the continuous learning feature of machine learning, systems can receive feedback and adjust recommendations based on real-time data analysis.
- Integration: Machine learning can integrate with other farm operations, making fertilization part of a holistic farm management plan.
Undoubtedly, the convenience offered by such a technology is unmatched. With Internet of Things (IoT) devices planting fertilizers based on machine learning recommendations, it’s like having a personal agronomist for every field.
Apart from accuracy, consistency is another major selling point of using machine learning in precision fertilization.
Human error is practically eliminated in this setup. Farmers achieve improved efficiencies through the elimination of redundant tasks and better decision-making. This consistent application of the appropriate fertilizer, at the right amount, to the correct location is invaluable in maximizing yields.
Moreover, machine learning algorithms can help make precision fertilization even more profitable. By factoring in the cost of different fertilizers, they can suggest ways to optimize farm expenditures while maintaining high yields, thus, becoming an essential tool for sustainable and cost-effective farming.
Overall, machine learning for precision fertilization is a fine collaboration of AI and agriculture – a nod to the future where technology serves as the trusted partner in food production.
4. Accurate AI-driven soil analysis for better yields
In Short: Artificial intelligence is revolutionizing farming through accurate, efficient soil analysis methods, informing crucial decisions on planting, fertilizing, and irrigation. Advanced AI technology predicts nutrient, pH, moisture levels and the physical properties of soil, resulting in more precise farming practices and significantly improved crop yields.
One of the most transformative ways AI technology is being utilized in improved crop yield is through accurate AI-driven soil analysis .
As any farmer will tell you, soil health is crucial in determining the productivity of a farm.
The composition of soil not only influences what crops can be grown but also impacts how well they grow.
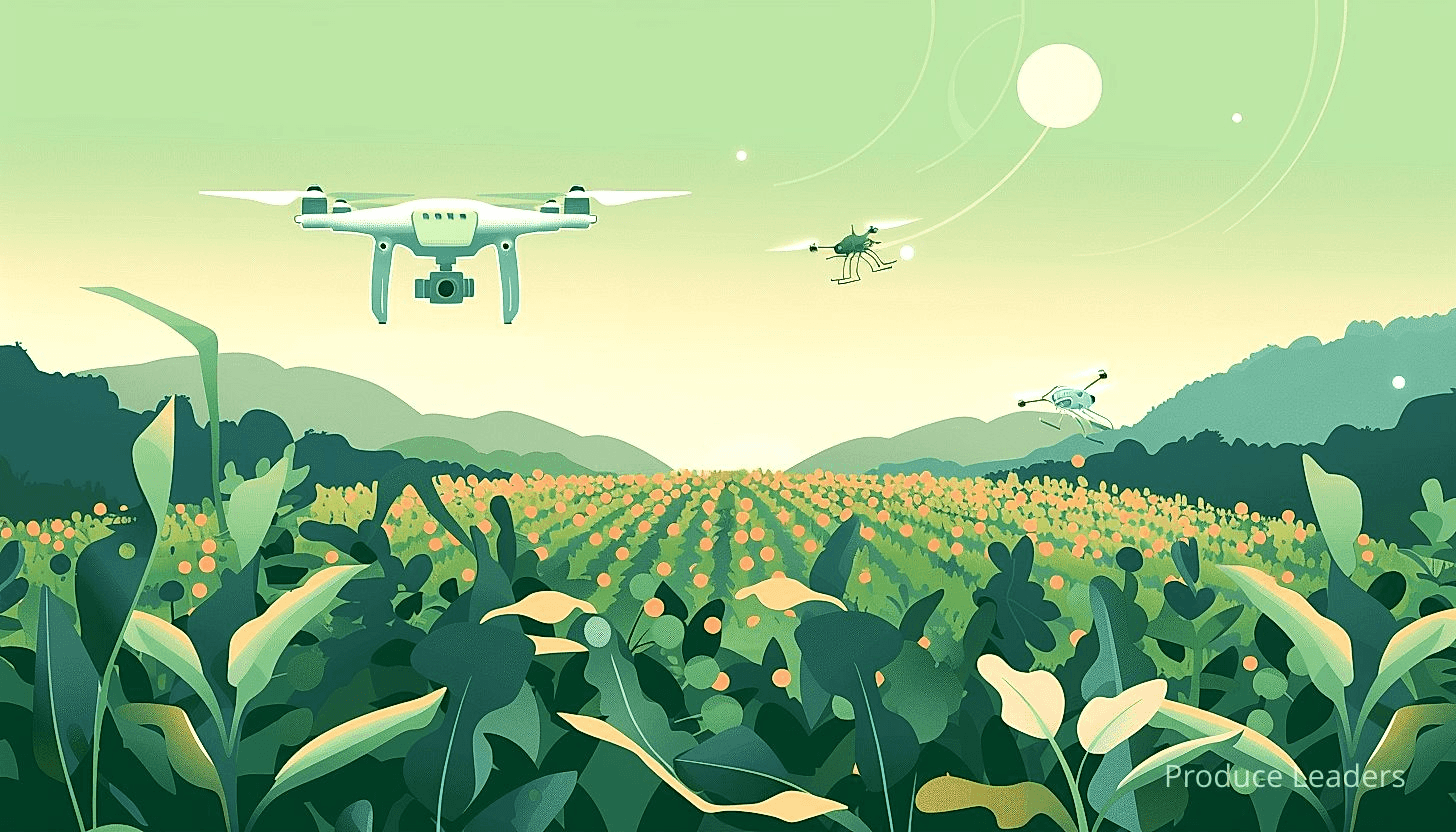
In traditional farming, determining the composition and health of soil was a labor-intensive and time-consuming process.
Collecting soil samples, sending them for laboratory analysis, and awaiting results involved significant costs in terms of both time and money.
Thanks to advances in machine learning and artificial intelligence, this process has become much faster and efficient .
Scientific methodologies are now being combined with artificial intelligence to scan and analyse soil samples.
Data collected from such AI-driven soil analysis technologies can be used to make informed decisions on planting, fertilizing, and irrigation, significantly increasing crop yields.
Here is a list of what AI-driven soil analysis can reveal about the soil:
- Nutrient levels: AI can determine the level of various nutrients present in the soil.
- pH levels: The artificial intelligence-based soil analysis can measure the acidity or alkalinity of the soil and predict its impact on crop growth.
- Moisture content: AI algorithms can measure how much moisture is present in the soil, which often determines if it is suitable for certain crop types.
- Structure and texture: The technology can identify the physical properties of the soil, including its structure and texture.
This highly advanced AI-driven soil analysis provides farmers with important information about their soil.
With this information, they can make more accurate decisions about which crops to plant, when to plant them, and how to manage their soil health.
As a result, crop yields are significantly improved .
Artificial intelligence is essentially transforming the scale and accuracy of soil analysis.
Detailed, precise, and quick soil analysis can lead to better decision-making and, ultimately, higher crop yields.
In this way, AI has become central to modern farming and plays a revolutionary role in soil analysis.
Beyond this, AI-driven soil analysis incorporates predictive analytics , which allows farmers to not only understand their current soil health but also predict future soil conditions.
This, in turn, can play a significant role in predicting potential yields and helping farmers plan accordingly.
Thus, through the power of AI, soil analysis has become not only faster, but also a powerful tool in increasing crop yields and planning future farming practices.
5. Predictive Analytics for Crop Diseases
In Short: Farmers worldwide use AI and machine learning to predict and manage crop diseases effectively, optimizing yields and sustainability. This approach, utilizing agronomic data, drone imagery, soil analysis and historical records, revolutionizes farming by mitigating disease risks and reducing reliance on pesticides, advancing global food production.
Farmers around the globe are harnessing the power of artificial intelligence (AI) to predict and prevent devastating crop diseases.
Thanks to advancements in AI and machine learning technology, we now have access to intelligent systems that can accurately predict the risk and spread of various diseases, thus helping to optimize crop yield.
This data aids in the prediction of disease outbreaks and assists farmers in taking preventive measures before yield loss ensues.
This method of predictive analysis is transforming traditional farming and bringing it to the forefront of technological advancement.
Inevitably, this has a significant impact on the agricultural industry, affecting everything from the economics of farming to agricultural sustainability and resource management.
There are a number of ways AI and predictive analytics can be used to tackle crop diseases.
- Firstly, machine learning algorithms can be trained to identify signs of disease in crops by analyzing drone or satellite imagery. This not only helps to catch diseases early but also reduces the need for harmful pesticides and chemicals.
- Secondly, predictive models can utilize historical data to anticipate future disease outbreaks, alarm farmers ahead of time, and enable them to take necessary steps to prevent spread.
- The use of AI for soil analysis can also aid in disease prevention. By assessing the health and nutrient status of the soil, farmers can adjust their cultivation methods accordingly.
This predictive approach is revolutionizing the agriculture industry and adding a new dimension to farming management.
As AI continues to advance, so too will its potential in disease control and crop yield maximization.
Furthermore, AI predictive analysis can help combat the effects of climate change on crops by anticipating changes in weather patterns or other environmental stressors that might increase disease vulnerability.
At the end of the day, the use of AI predictive analytics in disease control not only enhances crop yield but also promotes sustainable farming by reducing reliance on harmful pesticides and excessive use of resources.
This represents a major step forward in agricultural practices, one that will have long-term benefits for our ecosystem and food production globally.
Indeed, the future of farming lies in the augmentation of traditional techniques with smart, predictive technologies powered by AI.
6. Real-time crop growth monitoring with AI algorithms
In Short: The use of AI algorithms for real-time crop growth monitoring is revolutionizing agricultural practices by providing detailed insights into crop health and growth, allowing farmers to make immediate interventions. Utilizing technologies like sensors, machine learning and IoT, AI enhances farming efficiency, resource management and sustainability, while also supporting predictive analysis for improved crop yield.
The advent of AI algorithms has completely revolutionized the agricultural sector, especially in the realm of crop yield prediction. One such area that has seen significant progress is real-time crop growth monitoring. This technique is revolutionizing agricultural practices, providing significant benefits for both farmers and the environment.
Traditionally, farmers relied heavily on their knowledge, experience, and intuition for crop growth monitoring. However, this method is time-consuming, labor-intensive, and often inaccurate.
With the integration of AI algorithms, farmers can now monitor crop growth in real-time, allowing for instant adjustments and interventions when necessary. This not only enhances efficiency but also moves agriculture towards sustainability.
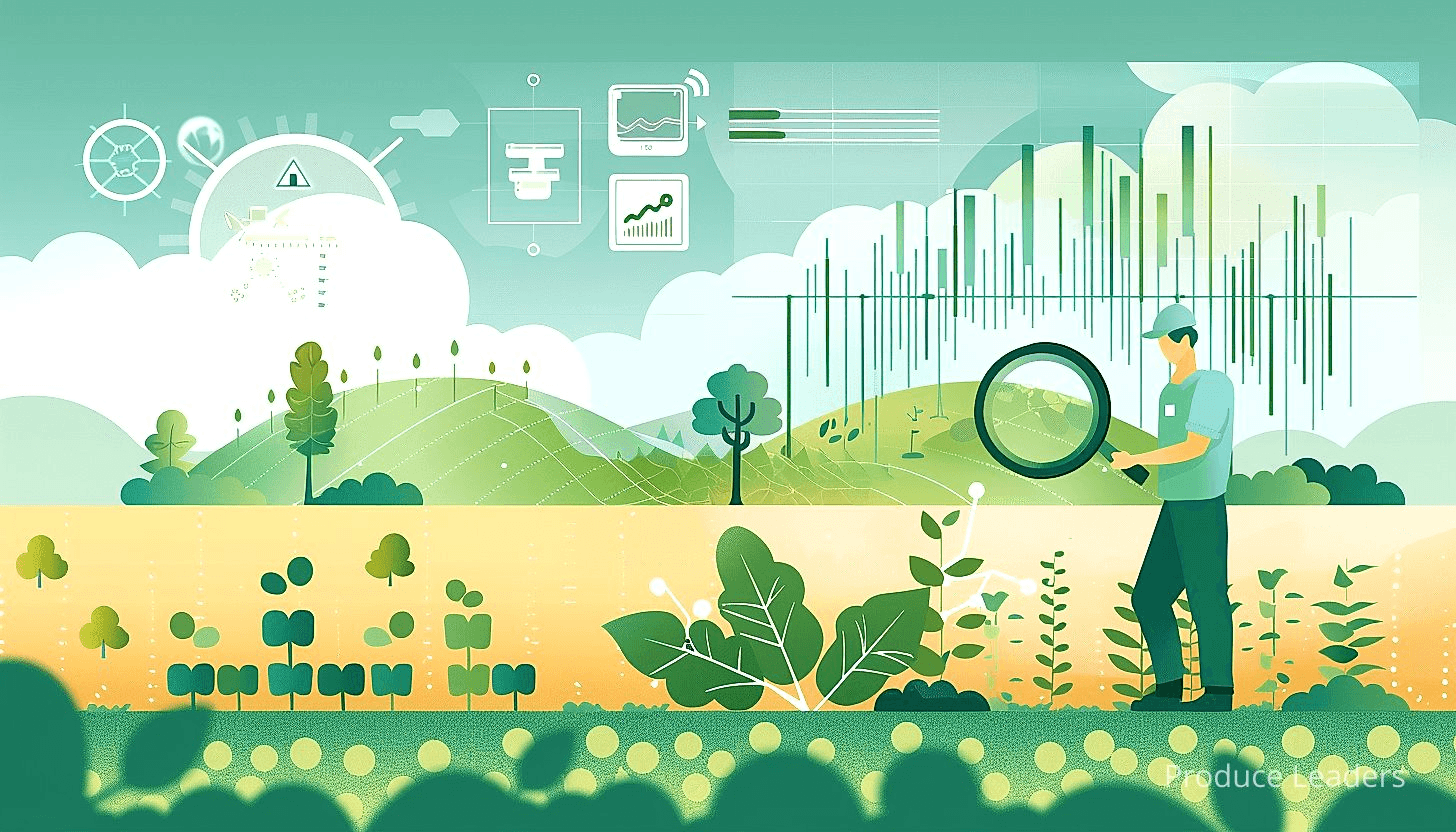
One of the ways AI accomplishes this is through image analysis. Cameras and sensors equipped on drones or other types of farming machinery capture high-resolution images of the crops. These images are then processed in real time, providing valuable information about the health and growth of the crops.
This real-time data can help farmers quickly identify any potential problems such as disease outbreaks, insect infestation, nutrient deficiency, or adverse weather damage. As a result, farmers can take immediate action to rectify these problems before they spread or cause irreparable damage.
Before we tell you more about AI’s role, it’s important to identify some key technologies that facilitate real-time crop growth monitoring. These include:
- Sensors: These gather real-time data regarding soil conditions, weather changes, and plant health.
- Machine learning: This technology helps to process the received data and make accurate predictions.
- Internet of Things (IoT): IoT devices allow automated control of farming equipment based on the processed data.
The AI algorithms, by processing the data from these technologies, can predict the crop growth patterns accurately. This allows farmers to calculate the best time for fertilization, irrigation, or harvest. These data-driven decisions can significantly increase the overall crop yield while consuming minimal resources.
Another incredible application of AI algorithms in real-time crop monitoring is that they can measure the growth of each plant individually. By analyzing the images of each plant, the AI can assess its individual health and growth rate. This is a level of precision that was impossible to be achieved with traditional methods.
One significant advantage of this precise and real-time crop monitoring is the optimization of resources. By receiving accurate data about the health and needs of crops, farmers can manage their resources more efficiently. This avoids over-irrigation, excessive use of fertilizers, and unnecessary manual labor, leading to cost-effective and sustainable farming.
Pro Tip: Incorporate AI algorithms in your agricultural practices to enable real-time crop growth monitoring, detect potential issues early, optimize resource use, and make informed data-driven decisions for enhanced crop yield and sustainability.
Furthermore, AI algorithms can also provide farmers with predictive analysis based on the constantly changing variables such as weather conditions, soil health, and plant growth metrics. This allows farmers to make informed decisions ahead of time, resulting in enhanced crop yield.
The innovative use of AI for real-time crop growth monitoring heralds a new era in agriculture. It is a powerful tool that offers a slew of benefits, from resource management, cost reduction, to improved crop yield and quality. Therefore, the adoption and integration of AI in crop growth monitoring will continue to be pivotal in the future development of sustainable agriculture.
7. Automating Weed Detection Using AI
In Short: Artificial intelligence is offering a novel solution to the persistent weed infestation issue in agriculture through automated weed detection. Drones equipped with AI can identify weed growth in fields quickly and accurately, enabling targeted herbicide use and reducing costs, while enhancing productivity on both large-scale and small-scale farms.
One foremost challenge in modern agriculture is the persistent issue of weed infestations that plague our crops.
These weeds compete with the crops for essential nutrients and sunlight, leading to reduced growth and following loss in yield.
Modern farming techniques have employed various methods of weed control, but most of them are labor-intensive, expensive and partially effective.
Artificial intelligence, however, promises a novel, efficient solution to this pestering issue through the automation of weed detection.
Drones equipped with high-resolution cameras and AI technology are able to maturely inspect fields and identify weed growths among crop plants.
These machines utilize advanced machine learning algorithms that can distinguish between plant species based on properties such as leaf shape, color, and size.
The following points highlight some of the unique factors that give AI-driven weed detection an edge over traditional methods:
- Real-time Detection – While it may take days for a human inspector to trudge through a large farm field to spot weeds, an autonomous drone can do it in a fraction of the time.
- Accuracy – Human detection methods can be prone to errors, but the machine learning algorithms used in AI detection have high rates of accuracy, up to 95% in some instances.
- Efficiency – By distinguishing crop plants from weeds accurately, AI allows for targeted use of herbicides, minimizing costs and environmental impact.
Following detection, these systems can either relay information to the farmers for further action or directly engage the identified weeds using precise application of herbicides or mechanical means.
This direct interaction reduces overall herbicide usage, cutting down costs and minimising the environmental footprint of the farming operation.
The applications of AI in weed detection are not limited to large-scale agriculture operations alone.
They can also be used in smaller farms and even home gardens, helping enhance productivity while significantly reducing labor.
AI-driven weed detection is a significant step towards precision agriculture, thus aligning with the continually growing demands for sustainability in the food production industry.
However, while automated weed detection offers a promising solution, it is crucial to remember that its implementation, like any technology, is not without challenges.
The costs associated with deploying these AI-enabled systems may be a hurdle for small-scale farmers.
Moreover, while machine learning algorithms improve with time and usage, their initial training and fine-tuning can still take considerable time and technical expertise.
8. AI allows comprehensive field imagery and analysis
In Short: AI revolutionizes agriculture with comprehensive field imagery and analysis, aiding in complex decision-making processes. Its functions include soil health analysis, disease detection, and weed management for optimized farming and forecasting models to anticipate future issues.
The world of agriculture stands on the brink of a revolution due to Artificial Intelligence (AI). AI’s wide-reaching potential incorporates comprehensive field imagery and analysis, a critical driver for burgeoning precision agriculture techniques.
Modern farmers are faced with a myriad of complex decisions each day, many of which depend on factors beyond their control, such as weather variables, soil conditions, and pests. This is where AI comes in, offering unprecedented capabilities to capture and analyze field data, thereby influencing decision-making.
Field imagery, acquired through advanced satellites or drones, can be processed by AI to monitor plant health, crop density or weed presence. This real-time and precise data allows farmers to make the best possible management choices at any given time.
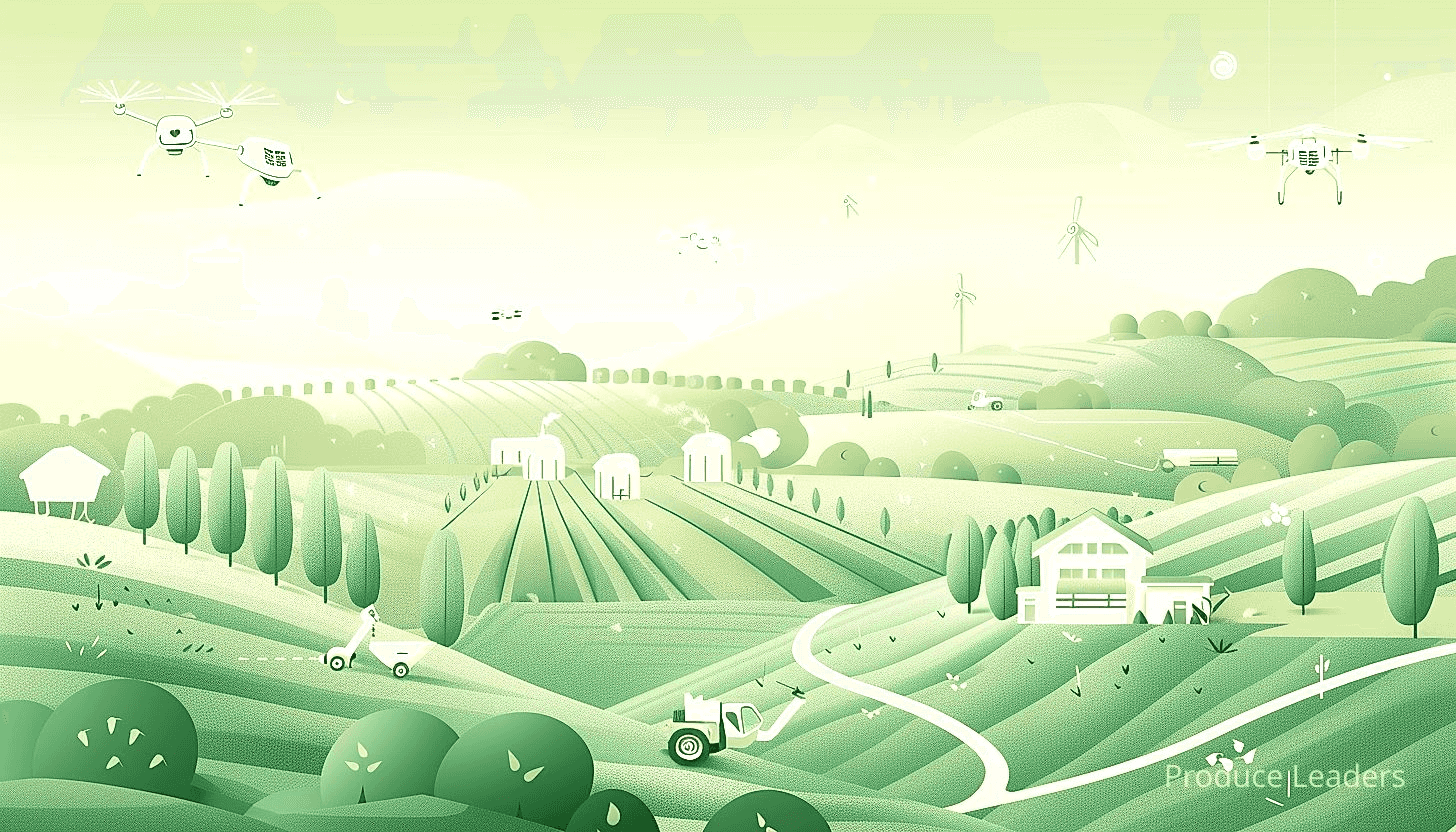
Moreover, this exhaustive analysis provided by AI can be used for different purposes. For instance, by recognizing patterns in the images, AI algorithms can identify the variables that most influence crop health and yield.
Now, let’s dig in into other ways comprehensive field imagery and analysis can boost crop yield:
- Soil Health Analysis: By inspecting the images, AI can identify the soil’s health, including its nutrient levels, moisture content or pH balance, to determine the best agricultural practices.
- Disease Detection: AI algorithms can identify early signs of plant diseases, helping farmers to react accordingly and limit potential damage.
- Weed Management: Identifying weeds in the images helps to optimize herbicide usage and can lead to more cost-effective and sustainable farming practices.
By analyzing a broad spectrum of data, the AI not only produces accurate results but also creates predictive models. This empowers farmers to anticipate potential issues by using these AI-driven forecasts, supporting proactive management strategies.
Moreover, the use of AI provides an undeniable efficiency bonus. The sheer volume of data that can be collected and processed far exceeds what any human could manage, thus, accelerating decision-making processes.
However, it’s important to remember that the implementation and use of AI technologies do require investment both in terms of finances and time. Advanced equipment must be purchased and the staff needs to be trained to use it. But the potential benefits of AI in field imagery and analysis ultimately outbalance the initial costs in many cases.
Important: AI’s potential in agriculture incorporates comprehensive field imagery and analysis, capturing and analyzing data to influence decision-making, monitor plant health, and identify variables affecting crop health and yield, ultimately accelerating decision-making processes and leading to more efficient farming practices.
Furthermore, despite AI’s transformative potential, it does not diminish the importance of a farmer’s experience and intuition. Yes, AI can definitely help interpret the imagery data and recommend actions but the final decision is still made by the farmers themselves.
At the end of the day, AI-driven field imagery and analysis are not far-fetched concepts of science fiction. They are increasingly becoming a reality, leading to more efficient, sustainable, and profitable farming practices. These tools allow farmers to harness the power of data and technology, helping them to meet the growing worldwide demand for food.
9. Optimizing Harvest Time through AI Models
In Short: AI in agriculture enables optimization of harvest periods by creating intricate models to predict the best times for harvest, factoring in elements such as crop type, growth stage, disease susceptibility, and projected market prices. These data-driven models are crucial to maximize farm productivity, minimize food waste and can revolutionize the future of farming by addressing global sustainability challenges.
One of the groundbreaking applications of artificial intelligence (AI) in agriculture is the optimization of the harvest period using sophisticated AI models.
This innovative approach revolves around the creation of precise models that can predict the optimal time for harvesting crops based on numerous factors.
By utilizing vast amounts of data and complex algorithms, these AI models can analyze and interpret patterns that would otherwise be impossible for a human to decipher.
Through this, AI models are capable of providing highly accurate predictions about when crops will reach their peak ripeness, thereby reducing wastage and boosting farm productivity.
One of the significant factors that these AI models take into account is the weather.
AI models can access and analyze weather data from various sources to estimate how different weather conditions will affect the growth and ripeness of the crops.
This analysis allows the models to provide precise predictions and recommendations about the best time to start harvesting each crop.
In addition to weather conditions, AI models also consider a wide range of other factors for optimizing the harvest time.
These include the crop’s type, growth stage, disease susceptibility, and projected market prices.
Let’s take a look at some of the common factors AI models take into account when predicting the optimal harvest time:
- Type of the crops: Different crops ripen at different times and under different conditions.
- Growth stage: AI models can predict when a crop will be at its peak ripeness based on its growth stage.
- Disease susceptibility: Crops that are susceptible to certain diseases >usually need to be harvested earlier to prevent crop loss.
- Market prices: AI models can also take into account predicted market prices when determining the optimal harvest time.
Through the integration of these factors, AI models can provide a comprehensive analysis and precise prediction of the optimal harvest time.
This AI-driven approach has shown great potential in maximizing the efficiency of the harvest process and ensuring that farmers get the most out of their crops.
Moreover, by leveraging AI technology, it is expected that we can address the global challenges related to food waste and ensure food security.
Therefore, AI’s role in optimizing harvest time represents a crucial step towards meeting the agricultural demands of the 21st century.
What’s importantly intriguing is, the more data these AI models can access and learn from, the more accurate their predictions become over time.
This underscores the transformative power of AI in agricultural practices and its crucial role in shaping the future of farming.
10. Machine Learning Models for Crop Yield Forecasting
In Short: Machine learning models are being utilized in agriculture to accurately forecast crop yield by using historical data and constant refinement. The use of these models, provided the data used is precise and accurate, is transforming traditional farming into more efficient and sustainable practices, by allowing for anticipation of outcomes and better decision-making.
Accurate crop yield forecasting forms an integral part of successful farming practices, ensuring that farmers are well-prepared to maximize yield output.
With the advent of Artificial Intelligence (AI) in agriculture, we now see the incorporation of Machine Learning (ML) models in forecasting crop yield.
These models, with the right data, can predict yield with remarkable accuracy, thereby redefining the scope and approach of farming.
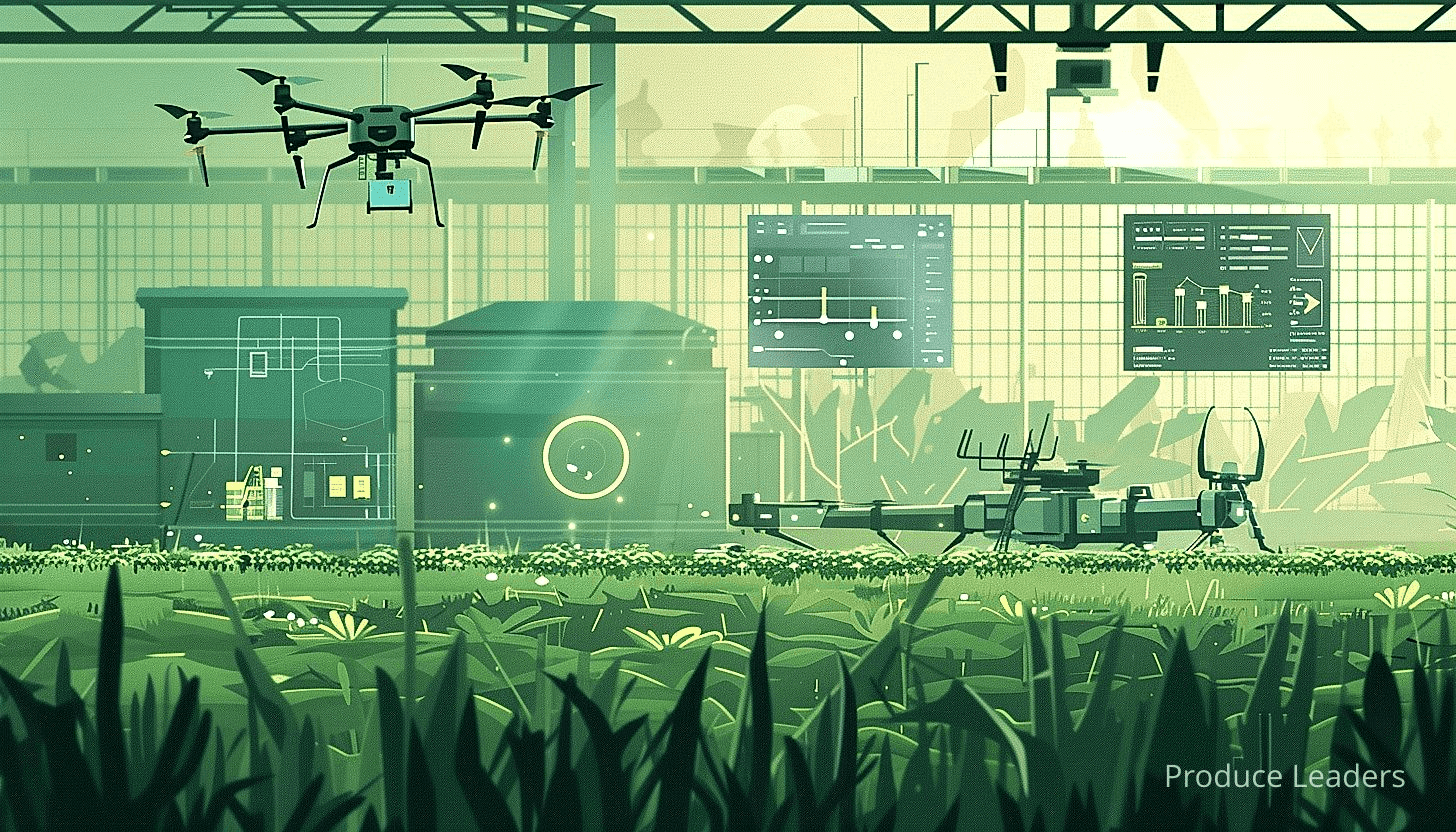
Machine learning models bank on the analysis of historical data.
This data gleaned from previous years’ yield, weather conditions, crop sequences, and farming techniques serve to train these models to predict future outcomes.
Agricultural researchers and AI engineering teams progressively feed data into the model to continuously improve its predictability.
Next, we will briefly explore some of these crucial data types used in Machine Learning models for crop yield forecasting.
- Weather data: This includes parameters like temperature, humidity, rainfall, and sunlight duration that significantly influence crop growth.
- Soil data: The type of soil, its fertility level, and the balance of minerals all impact the crop yield, and hence this information is paramount.
- Crop data: The unique requirements and growth conditions of individual crops are an essential input to the model.
Through constant learning and refinement, these models become better equipped to make accurate predictions contributing to informed decision-making in farming.
More significantly, Machine Learning models can unearth patterns and correlations that might not be obvious to the human eye.
For instance, the model might find a surprising correlation between a specific wind pattern or humidity level and the impact on crop yield.
It’s the complexity of these models that actually finds patterns unanticipated by humans, thereby aiding in yield optimization.
Machine Learning models for crop yield forecasting are, therefore, transforming the agricultural landscape, shaping a new era of precision farming.
However, the effectiveness of these models largely depends on the accuracy and quality of the data input.
Irregular or incorrect data can lead the model to false predictions.
Thus, it’s crucial that the data used to train the model is as precise and accurate as possible.
Pro Tip: Ensure to feed precise and accurate data into Machine Learning models for crop yield forecasting to avoid false predictions and maximize farming output.
Integrating AI with agriculture, especially in the form of Machine Learning models for crop yield forecasting, epitomizes the embracing of technology for sustainable and efficient farming practices.
It’s undoubtedly a significant stride towards marrying traditional agricultural wisdom with contemporary technological innovation.
The Bottom Line
AI is clearly revolutionizing the agricultural sector, particularly, in the area of crop yield prediction. Through advanced analytics, predictive modeling, multi-spectral analysis and machine learning, AI is helping the farmers to make data-based decisions, boost productivity and increase their overall returns. The transformative potential of AI doesn’t just reside in its ability to predict accurately, but also in how it can be used to foresee and ward off crop diseases and pests, ensuring a healthier harvest. AI systems also have the capacity to process vast data repositories rapidly, which provides a more interactive and real-time forecast helping farmers in proactive decision-making. Thus, farmers and agriculturalists should embrace AI technologies to enhance crop yields, making farming more sustainable and profitable in the long run.